Introduction
Predictive sales analytics for food and beverage is changing. It uses past sales info to guess future sales so businesses can see customer trends and improve their work processes. This helps companies better understand what customers want and manage their stock more efficiently.
Key Tools in Predictive Sales Analytics for Food & Beverage
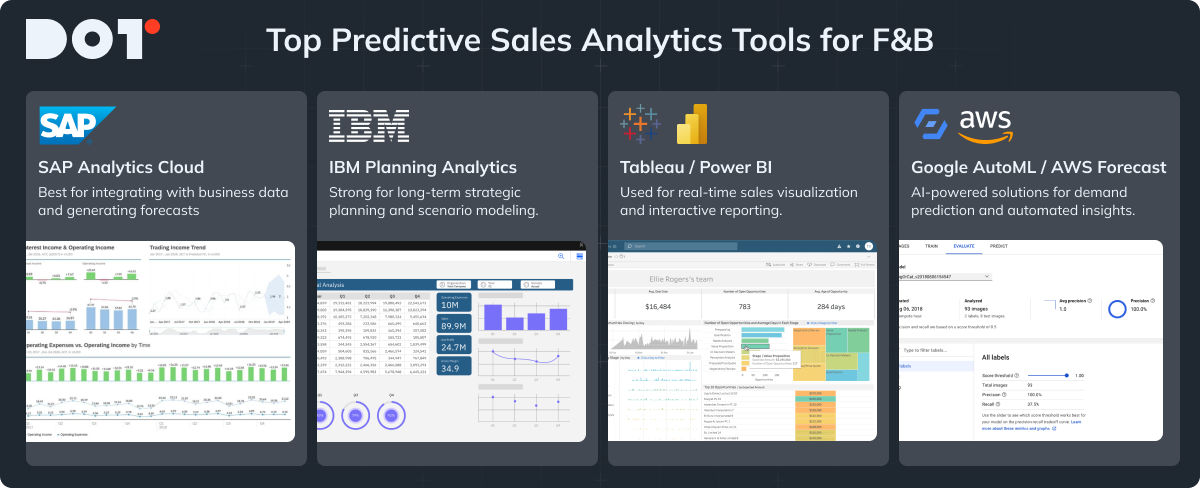
Detailed Exploration of Predictive Modeling and Sales Forecasting Tools
In the food and beverage world, tools for predictive modeling and sales forecasting are super important. Tools like SAP Analytics Cloud and IBM Planning Analytics are very popular because they are great with big data and give accurate predictions. SAP Analytics Cloud works well with existing data, while IBM Planning Analytics is good at making precise long-term plans. Predictive sales analytics for food beverage empowers these tools to transform historical data into actionable insights.
These platforms also let businesses plan scenarios by using imagined data to see different sales results. This helps in knowing what could happen if, for example, new products are launched or prices change. They often have charts and graphs that make the data easy to understand, helping businesses make smart, clear decisions.
Analysis of Machine Learning Algorithms Specifically Suited for Sales Predictions in the Food and Beverage Sector
Machine learning is key to finetuning sales forecasts in food and beverage. Common algorithms like regression analysis, time series models, and decision trees are very useful. Regression analysis finds out how things like pricing and advertising affect sales. By knowing these links, companies can see how changes might affect sales.
Time series models, like ARIMA, help understand data that changes over time. They find trends and patterns, helping businesses adjust to changes in demand. Decision trees make decision-making easy by showing different possible outcomes clearly. This way, businesses make complex choices easier.
Understanding Consumer Behavior with Predictive Analytics
Explanation of Data-Driven Insights into Consumer Preferences Using Predictive Sales Analytics
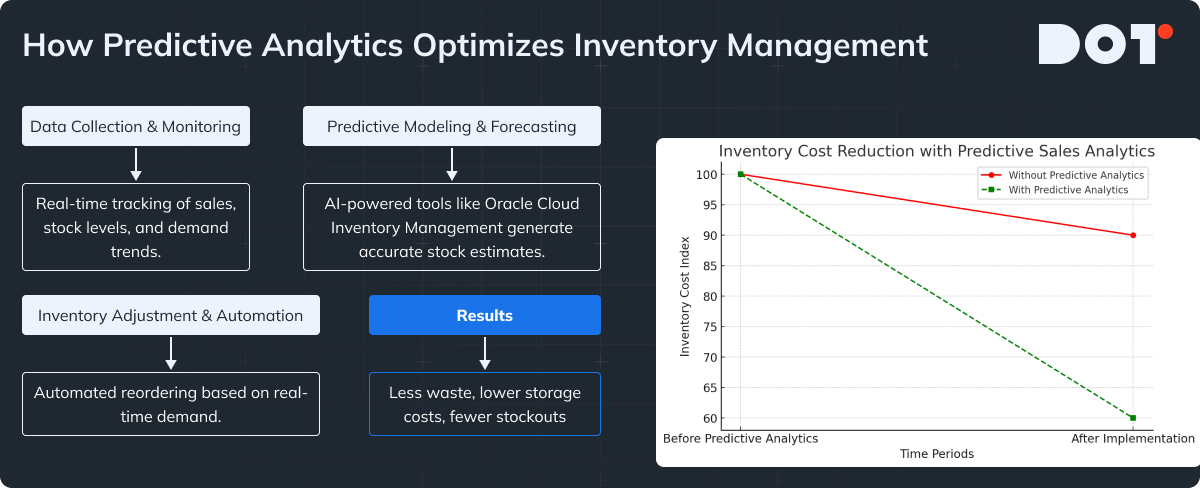
Predictive analytics dives deep into what customers like by looking at their past buying habits. Data like what people buy, how often, and which products they like reveal patterns. One technique, called clustering, groups customers with similar behaviors together. Knowing these groups helps make marketing that hits the mark.
For instance, we might find health lovers, bargain hunters, and foodies. By speaking directly to these groups—like discounts on organic foods or bundled deals—businesses connect better. If you’re curious about how this applies to your business, our Dot Analytics experts are ready to chat! Consumer behavior analysis in the beverage market provides critical insights into developing targeted marketing strategies.
Practical Steps to Analyze Purchase Patterns and Seasonal Influences in the Food and Beverage Market
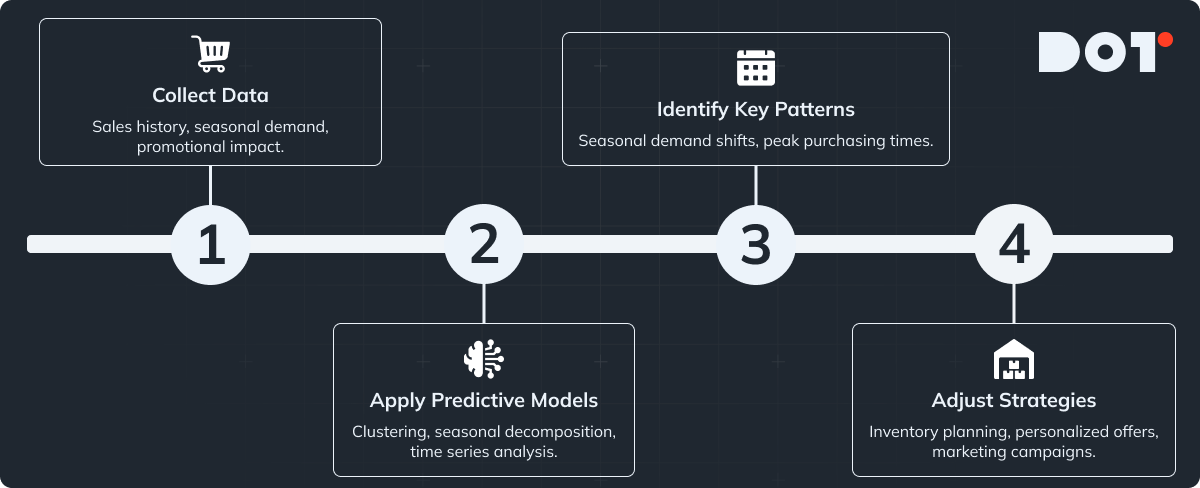
Looking at purchase patterns in food and drinks means using time, ad campaigns, and economic info to get the big picture. Seasonality majorly influences buying trends. Using a method called seasonal decomposition helps see the big picture by breaking data into trends, season patterns, and leftovers.
- Seasonal factors often drive sales, like more ice cream in summer or hot drinks in winter.
- By seeing these patterns, businesses can adjust stock and marketing.
- Past data is useful for predicting these patterns and planning production and delivery.
Need tips for your business? Dot Analytics experts are just a call away!
Optimizing Inventory Management with Predictive Sales Analytics for Food & Beverage
Methods to Synchronize Inventory Levels with Sales Predictions
Managing inventory well means matching stock with sales forecasts. This involves linking inventory systems to predictive analytics for real-time views and estimates. This helps businesses keep the right amount of stock and avoid running out or having too much.
Systems like Oracle Cloud Inventory Management offer wide solutions, from order to supply chain logistics. They suggest reorders based on predictive plans, aligning stock with demand. This reduces carrying costs and helps manage cash flow.
Real-Time Data Utilization to Prevent Overstocking and Stockouts in the Food Industry
Real-time data is crucial to preventing overstock and stockouts in the food industry. With IoT and sensors, businesses get continuous data on stock and sales, building a responsive supply chain. This tech adjusts orders automatically based on current demand insights.
Software constantly analyses data, predicting future stock needs accurately. This allows businesses to quickly react to changes in demand, whether seasonal or unexpected. Improving inventory management also cuts down on waste from expired goods. Understanding predictive sales analytics for food beverage is critical to overcoming these challenges.
Types of Data for Effective Implementation of Predictive Sales Analytics in Food & Beverage
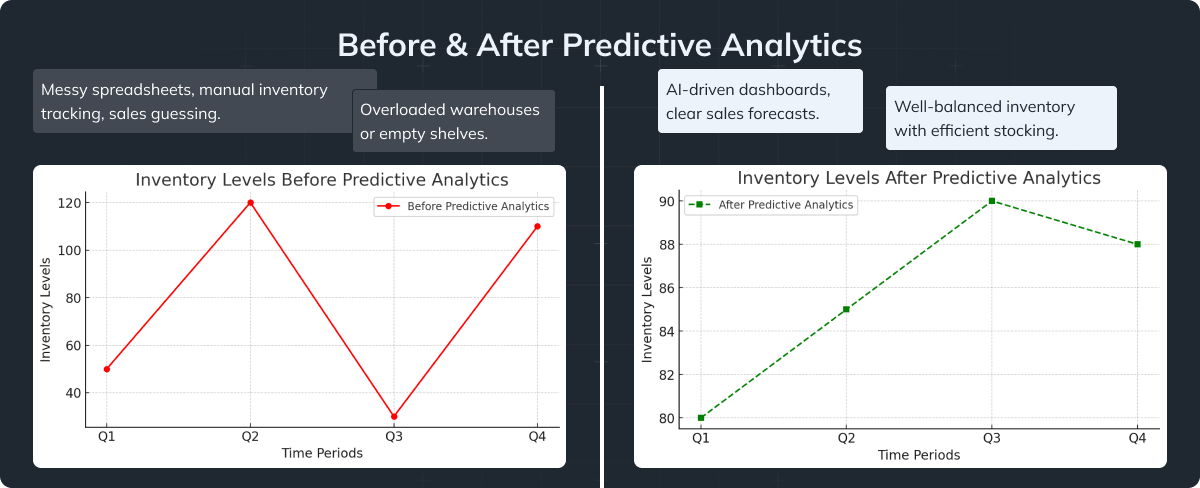
Essential Data Categories: Transactional, Behavioral, and Demographic Data
To successfully use predictive sales analytics, gathering and analyzing three main data types is key: transactional, behavioral, and demographic. Transactional data tracks sales transactions, revealing buying trends over time. It helps identify purchase patterns, pricing, and order frequency.
- Behavioral data looks at what affects buying decisions and how often customers return.
- It refines marketing strategies for targeted consumers.
- Demographic data provides background essentials like age, income, and location, informing strategic approaches.
Techniques for Ensuring Data Quality and Security in the Analytics Process
Good quality data ensures accurate predictions. Data cleaning involves checks to remove duplicates, correct mistakes, and fill in gaps. This data reliability is crucial for sound business predictions. Data security is equally important, using encryption to ensure privacy and comply with regulations.
Robust cybersecurity measures ensure ethical data use and protect consumer info, including regular audits, firewalls, and access controls. This combination of quality and security supports strong predictive analytics. Sales trend analysis in beverages can inform better marketing and distribution strategies, helping businesses respond swiftly to changing preferences.
Implementing Predictive Sales Analytics for Food Beverage
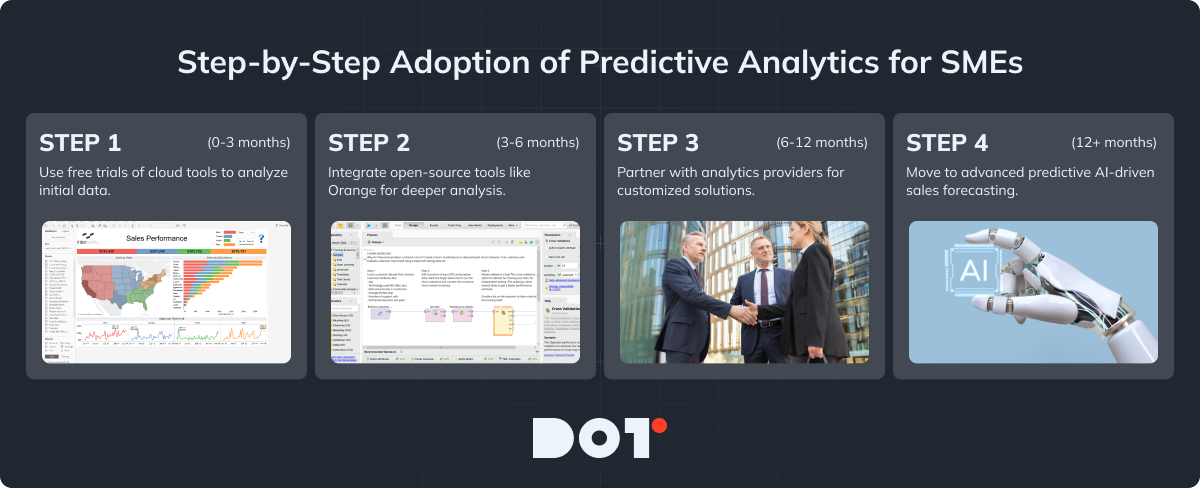
Cost-Effective Tools and Solutions for Small and Medium Enterprises
Small and medium-sized businesses can start using predictive analytics without spending much by choosing cloud services and open-source software. Platforms like Amazon Forecast offer flexible and affordable solutions, ideal for companies starting with analytics. They’re a great start because they can handle various data amounts.
SMEs often start small, with basic models, and get more advanced as they grow in data skills. This step-by-step approach helps businesses gain comfort and experience with analytics, gradually moving to complex tools as they need. Predictive sales analytics for food beverage can redefine how SMEs approach market challenges and opportunities.
Open-source software like Orange and RapidMiner provides strong predictive capabilities at low cost.
Strategies for Gradual Integration Without Significant Upfront Investment
To slowly introduce predictive analytics, businesses can integrate models into current processes. Partnering with analytics providers offering tiered services lets companies try innovation without a big spend. Free trials help businesses test before investing in comprehensive analytics.
As SMEs bring analytics into their operations slowly, they gain insights that set up for advanced applications later. By checking fitting capabilities well, businesses can choose solutions seamlessly fitting their current setups, minimizing disruption, and maximizing returns.
Q&A with Dot Analytics. Predictive Sales Analytics for Food & Beverage.
What is predictive sales analytics, and how does it work for a food and beverage business?
Imagine you run a cafe or a restaurant—predictive sales analytics means using your past sales data to get a clear picture of what might happen next. For example, if you notice that latte sales spike on rainy days or during morning hours, you can prepare by adjusting your staffing, inventory, and promotions accordingly. It’s about turning your historical numbers into practical forecasts that help you plan better day-to-day.
What kind of benefits can I expect—like smoother inventory management and happier customers?
When you use predictive analytics, you can fine-tune your inventory so that you have just the right amount of stock at the right time. Think about avoiding wasted food because you overbought, or never running out of a popular item during a busy weekend. Plus, by knowing what your customers love, you can tailor promotions and menu items to keep them coming back—leading to happier customers and improved sales.
Which tools and platforms should I be using to forecast sales in this industry?
There are a lot of great tools out there. For robust needs, platforms like SAP Analytics Cloud or IBM Planning Analytics handle large volumes of data and can give very detailed forecasts. If you’re just starting out or on a tighter budget, open-source options like Apache Spark or even cloud services like Amazon Forecast can be effective. These tools help you process your sales data and create models that predict future trends based on your unique business environment.
How can predictive analytics help me manage seasonal trends and shifting customer preferences?
By digging into your historical data, you can uncover seasonal patterns—like a surge in smoothie sales during the summer or higher demand for hearty soups in winter. This insight lets you adjust your stock levels and marketing campaigns in advance. It’s like having a weather forecast for your sales, so you can always be prepared for changes in customer behavior.
What types of data should I collect to ensure my sales predictions are accurate?
To get the best forecasts, you’ll want a mix of data. Transactional data (like sales receipts) tells you what’s been sold and when. Behavioral data (like website clicks or loyalty program interactions) shows how customers are engaging with your brand. And don’t forget demographic details—age, location, and other factors that influence buying habits. The more detailed your data, the clearer the picture becomes.
How do I add predictive analytics to my current operations without causing major disruptions?
Start small—perhaps run a pilot project in one area of your business, like a specific product line or location. Integrate the analytics gradually, so your team gets comfortable with the new insights before you roll them out company-wide. This phased approach minimizes disruption and allows you to tweak processes along the way.
What common challenges might I face when setting this up, and how can I overcome them?
You might deal with messy data, integration issues between different systems, or even a lack of technical know-how. A good starting point is cleaning up your data to ensure accuracy. Then, use flexible tools that can integrate with your existing systems. And if it feels overwhelming, partnering with experts can help guide you through the process step by step.
How do machine learning algorithms boost the accuracy of sales forecasts in this sector?
Machine learning models learn from your data over time. They can pick up on subtle patterns—like how a slight change in pricing or a special event impacts sales—that traditional methods might miss. By continuously adjusting based on new data, these algorithms make your forecasts more precise, helping you plan inventory and promotions with greater confidence.
How can I use these insights to fine-tune my pricing and promotional strategies?
Once you understand your sales patterns, you can experiment with different pricing strategies or promotional offers. For instance, if you see that a small discount significantly boosts sales during a slow season, you can plan targeted promotions to maximize revenue. Essentially, the insights help you test ideas, measure what works, and adjust your tactics for better results.
Conclusion
Predictive sales analytics is changing the food and beverage sector. With advanced modeling and forecasting tools, companies predict future trends, shape marketing, and optimize stock. SAP Analytics Cloud and IBM Planning Analytics are key platforms aiding these processes with deep insights.