Introduction
Organizations need to manage their data well to use it effectively. Two main ways to store data are data lakes and data warehouses. These two have different purposes, and it can be not very clear to choose between them. Engaging in dwh consulting can help clarify your options. Additionally, many organizations find that seeking data lake consulting provides them additional insights into managing their data better. This article will explain the differences between data lakes and data warehouses, how they are used, their costs, and how to pick the right one for your organization.
Key Differences between Data Lakes and Data Warehouses
Definitions and Objectives
A data lake is like a big ocean filled with all kinds of data. This data can be clean data, like numbers in a spreadsheet, or messy data, like social media posts. Organizations can collect a lot of data quickly because it doesn’t need to be processed first. The main purpose of a data lake is to allow quick collection of data for future analysis. This is important for businesses that gather various types of data often. For organizations exploring data management strategies, dwh consulting often proves beneficial in understanding these nuances. Moreover, implementing big data analytics can empower businesses to derive deeper insights from their data lakes.
On the other hand, a data warehouse is similar to a well-organized library. Here data is cleaned and stored in a clear way that makes it easy to find. The goal of a data warehouse is to provide reliable data for analysis to help make better business decisions. Before data can be stored in a warehouse, it must be cleaned, transformed, and organized properly. Additionally, firms using dwh consulting can improve their warehouse structures effectively, ensuring they meet evolving data architecture strategies.
Structural Differences
It’s important to understand how data lakes and warehouses are built:
- Data Lakes: These use a schema-on-read approach. This means that data is stored as it is, without needing to change its structure right away. The structure is applied when the data is analyzed, which allows for great flexibility. You can keep many types of data—text, videos, images—all in one place. For example, if a company collects data from users, it can store everything in its original form. Leveraging dwh consulting services can help organizations optimize this approach. Integrating cloud data management solutions can streamline the process of storing and analyzing diverse data sets.
- Data Warehouses: These use a schema-on-write approach. This means that data must be structured and formatted before it can be stored. Organizations need to think carefully about how to organize their data beforehand. This makes it easier to access and analyze data later. Engaging with experts in dwh consulting can greatly aid businesses during this planning phase, promoting more efficient data storage solutions.
Applications Across Different Sectors
- Data Lakes: These are very useful in fields like finance, helping quickly detect fraud by analyzing various types of data. In healthcare, data lakes can manage different patient information, giving better insights into care. The telecom industry uses data lakes to monitor networks by analyzing logs and performance. Organizations often turn to dwh consulting to gather insights on best practices tailored to their respective sectors.
- Data Warehouses: These are important in areas where organized data is crucial. Retailers use data warehouses for detailed sales reports to track marketing, inventory, and customer behavior. Businesses in cloud computing rely on data warehouses to analyze structured data for better efficiency. Moreover, professionals well-versed in dwh consulting can offer valuable guidance on implementing effective solutions, particularly in enhancing business intelligence consulting initiatives.
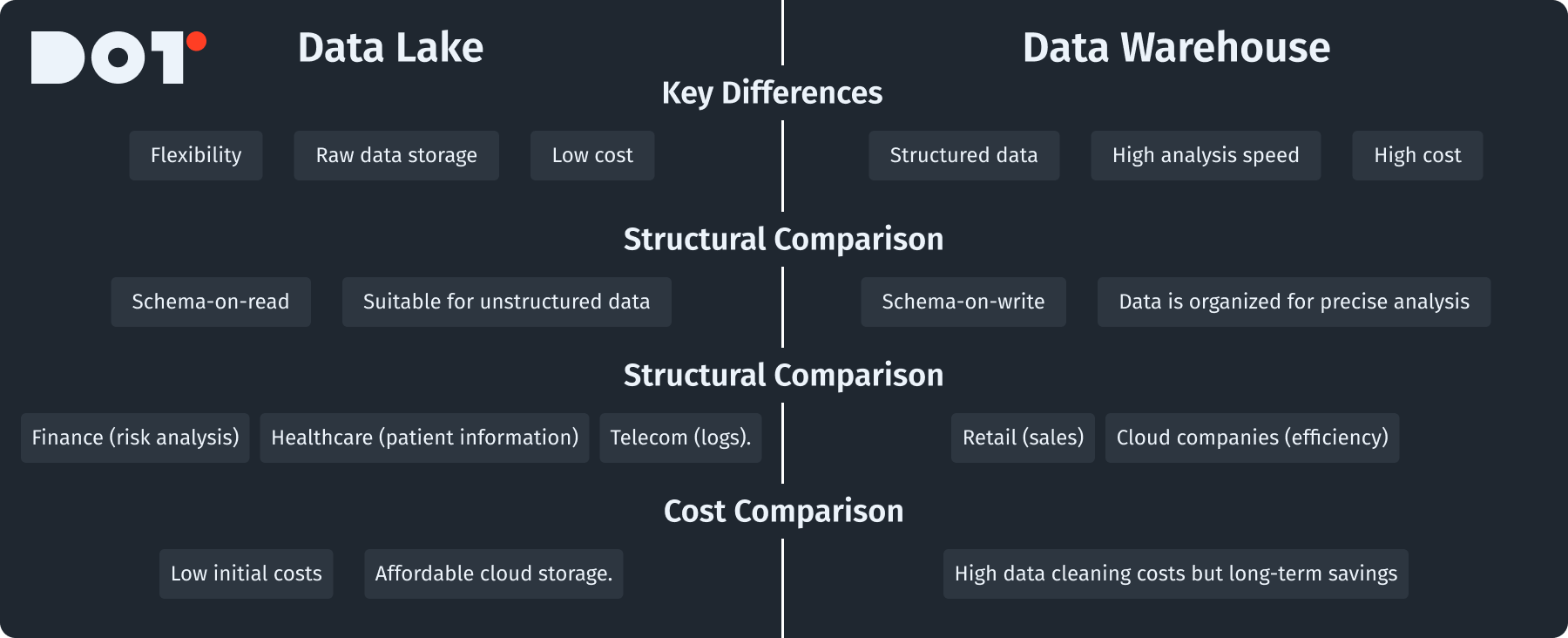
Expert Consultation
Choosing the right data storage solution is not easy. Companies often benefit from talking to experts who can help them make smart choices based on their own data management challenges. Expert help can answer questions about using data lakes and warehouses and share the best practices for your business. Whether you are just starting to look at options or want a complex system, reaching out to professionals can lead to better decisions regarding your data needs. Investing in dwh consulting can streamline this consultation process significantly. This approach can also help organizations tailor their strategies based on their specific needs for data lake consulting.
Cost-effectiveness of Data Storage Solutions
Cost Implications
When talking about costs, think about these points:
- Data Lake Costs: Data lakes can be cheaper, especially for large amounts of data. They can use affordable cloud storage options like AWS S3 or Azure Blob Storage, which help in saving money. Since you don’t need expensive transformations at the beginning, the ongoing costs might be lower.
- Data Warehouse Costs: Data warehouses tend to be more expensive for storage and upkeep. Because data has to be transformed and cleaned before being stored, organizations might spend more on these processes. They often need costly technologies to manage their structured data, leading to higher overall costs. Dwh consulting can provide detailed analysis that leads to cost-effective choices across these data storage solutions.
Long-term vs. Short-term Financial Impacts
While data lakes seem cheaper at first, it’s essential to think about future costs. Managing a data lake can bring ongoing expenses for data processing as the need for organization grows. On the other hand, although data warehouses require higher initial investments, they can have better long-term value. They provide accurate and well-organized data for decision-making, which can lead to more reliable insights—key advice obtained through dwh consulting conversations.
Case Study: Avand Medical’s Financial Assessment
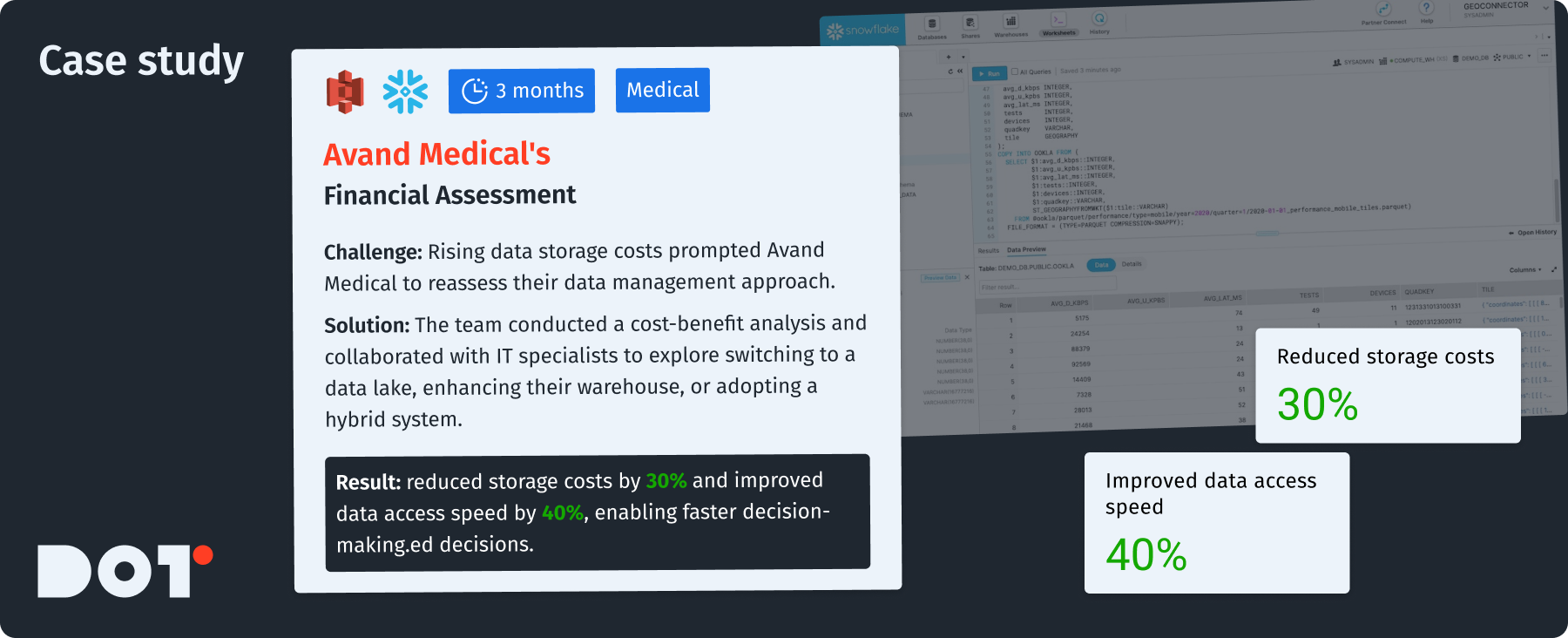
- Company Overview: Avand Medical creates innovative medical devices to improve patient care. They wanted to check their data storage costs to optimize spending.
- Challenge: Avand Medical faced rising costs in their data solutions and needed to closely evaluate their current data usage.
What They Did
The team aimed to find out whether to switch to a data lake, enhance their warehouse, or create a hybrid system.
How They Did It
- They performed a cost-benefit analysis to look at the operational costs of data storage.
- They worked with IT and data management specialists to identify necessary tools and data policies, including support from dwh consulting for advanced frameworks.
Team Composition: 3 data analysts and 2 IT experts
Project Duration: 3 months
Technologies Used: AWS S3 for the data lake prototype and Snowflake for the data warehouse \
Results: By strategically planning, Avand Medical cut their data storage costs by 30% and improved data access speed by 40%, making it easier for their teams to make decisions.
Types of Data to Store in a Data Lake vs. Warehouse
Suitable Data Characteristics
Understanding what type of data to store in which location is crucial:
Data Lakes are great for storing raw and semi-structured data, such as:
- Web data in formats like JSON and XML
- Log files for tracking real-time actions
- Unstructured data like audio, images, and videos
This allows businesses to use different kinds of data without needing to fit them into fixed formats at first. Dwh consulting can help identify which data types will yield the most benefits in different scenarios, especially when considering the integration of big data analytics tools.
Data Warehouses are meant for structured data, which include:
- Tabular data from systems like sales and inventories
- Cleaned data sets for reporting and business analysis
Structured data is organized and predictable, making it easier to generate reliable reports.
Use Case Scenarios
Here are some examples to clarify when to use a data lake or a warehouse:
- Data Lakes: A marketing team collecting data from social media and websites can use a data lake to store everything as-is. This means they can analyze how customers interact without needing to format the data in advance.
- Data Warehouses: A retail company evaluating its quarterly sales would use structured data from transaction systems (like point-of-sale systems). This data can be compiled into reports for executives who can then make strategic decisions based on historical data. Discussions with experts through dwh consulting can also clarify how to leverage these data types effectively. Integrating cloud data management solutions can enhance the performance of both systems.
Examples of Data Classification
Organizations categorize their data based on its purpose and use:
- In a data lake, the focus is on all data types for exploration, making it easier to identify patterns.
- In a data warehouse, data is categorized for specific functions, aligning closely with company goals.
Understanding these classifications can help organizations use both systems to get the most out of their data.
Understand the Impact
Optimizing Data Storage Costs
Choosing between a data lake and a data warehouse affects an organization’s efficiency and costs. Balancing short-term savings with long-term investments is vital. A data lake may offer immediate flexibility, but there can be upcoming costs when organizing the data later on. If you need fast insights from organized data, a data warehouse could save you money in the long run. Consulting through dwh consulting can give you the clarity needed on these trade-offs, and leveraging overall data storage solutions can provide organizations with better strategies.
Performance and Speed Comparisons
Ingestion and Retrieval Speeds
Speed is an important factor when comparing data lakes and warehouses:
- Data Lakes: They often allow for quicker data ingestion since they take different formats without needing to change anything right away. Organizations can see new data almost in real-time, which is great for capturing fresh information. Dwh consulting can help establish best practices for maximizing this benefit.
- Data Warehouses: These usually take longer for data ingestion because the data must be processed first. This extra step can cause delays, especially when fast reporting is crucial. Many organizations find it helpful to balance both systems to meet different speed requirements.
Evaluation of Query Performance
Another crucial comparison is query performance:
- Data Lakes: While they provide fast ingestion speeds, querying can be slower. Data is processed during the reading phase, so initial searches might take longer to complete.
- Data Warehouses: They provide faster query performance because data is already structured, making it easy to analyze quickly. This structured setup helps with immediate insights, which is important for business intelligence tasks. Companies can turn to dwh consulting for enhanced strategies around query performance, especially in leveraging business intelligence consulting.
Case Study: jmc’s Experience with Optimizing Performance
- Company Overview: jmc offers school management software for better administrative services, including tracking student data.
- Challenge: jmc faced slow performance with their current data warehouse, affecting their service.
What They Did
To improve their analytics speed, jmc decided to review their system.
How They Did It
- They set up a robust data lake for real-time data gathering, leading to faster data retrieval.
- They optimized their data warehouse to better manage structured data, greatly improving querying speed, with guidance from dwh consulting.
Team Composition: 4 data engineers and 2 application developers
Project Duration: 2 months
Technologies Used: Azure Data Lake for ingestion and Google BigQuery for data warehousing \
Results: jmc saw up to a 50% increase in data processing speeds, significantly improving their service delivery.
Tools and Technologies for Implementation
Popular Tools
Choosing the right tools for data lakes or warehouses depends on budget and needs:
- Data Lakes: Useful tools include:
- AWS S3: Ideal for scalable object storage for many data types.
- Azure Data Lake: Works well with Microsoft products for cloud storage.
- Google Cloud Storage: Good for scaling data storage quickly.
- Data Warehouses: Established options include:
- Snowflake: A cloud platform for structured data analysis.
- Amazon Redshift: Efficient for large-scale data analytics.
- Google BigQuery: Fast and serverless for analyzing large datasets deeply. Consulting on dwh could recommend the most appropriate technology stack for your organization, while a focus on data lake consulting can help tailor these solutions further.
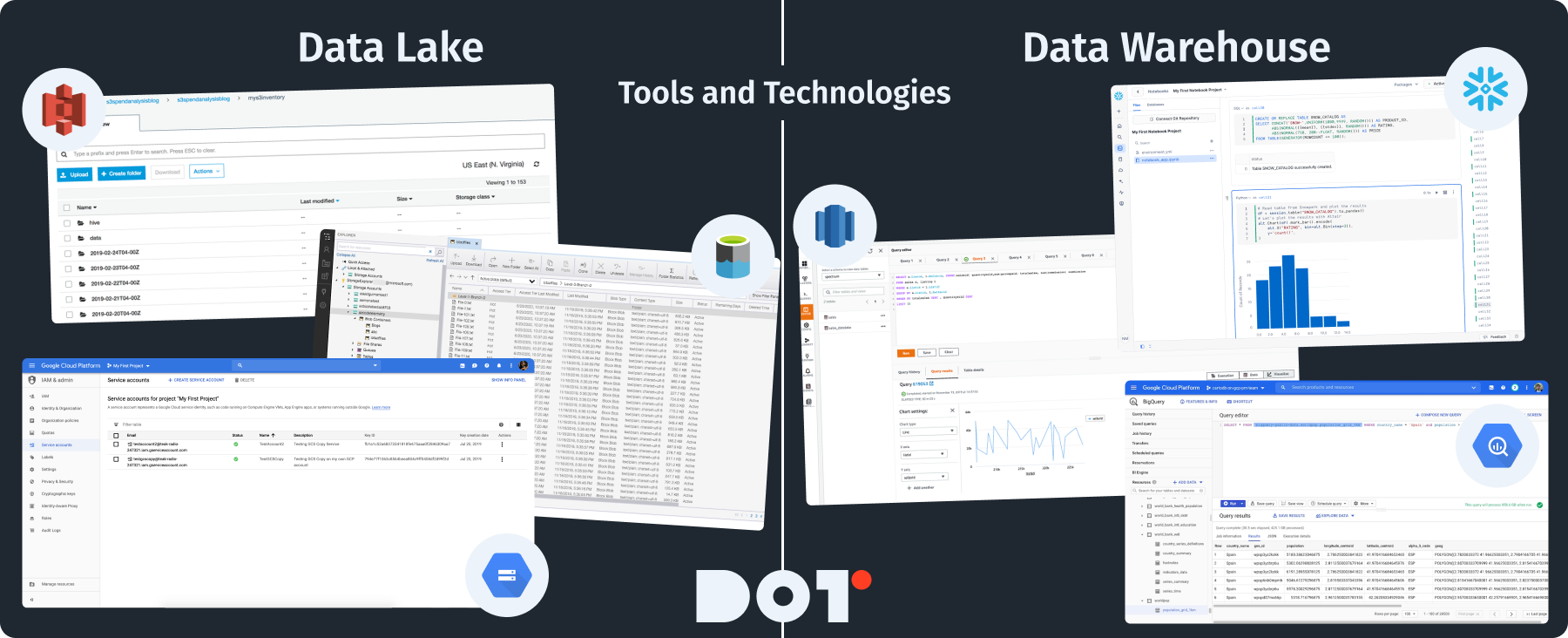
Integrating Existing Tools
Using existing tools with new data solutions can improve efficiency. If you already use ETL tools like Talend or Matillion, make sure they work well with your chosen data lake or warehouse. An effective data process can reduce duplication and save time. For example, if you’re using cloud storage, ensure your ETL tool automatically sends data to the right storage without much manual input.
Selecting the Right Technology Stack
Choosing the best technology involves knowing your organization’s needs:
- Evaluate scalability: Will the tools grow as your data increases?
- Integration capabilities: Do they fit into your current system without too much work?
- Analytics support: Choose tools that have built-in analytics or can link easily to business intelligence programs. Recommendations from dwh consulting can streamline this selection process tremendously, ensuring strong alignment with data architecture strategies.
Technology Assessment
Guidance on Choosing Tools
Choosing the right tools needs careful thought:
- Assess specific data needs: Look at current and future data volumes. Will there be more unstructured or structured data?
- Consider growth: Knowing how quickly data may expand can impact your choice.
- Align with governance policies: Ensure compliance with regulations as your data grows. Engaging with dwh consulting can help navigate these complexities.
Good data governance is critical, especially for sensitive information. If you’re concerned about this, book a free 20-minute consultation with a Dot Analytics expert.
Data Security Considerations
Varying Security Protocols
The security of data lakes and warehouses differs based on data types:
- Data Lakes: Focus on access controls to protect against security breaches. Security measures can depend on data sensitivity; for example, patient records need stricter measures than less sensitive data.
- Data Warehouses: Usually enforce stricter regulations because the data is more organized. Clear governance helps comply with laws like GDPR or HIPAA. Strategies for managing security can be tailored through dwh consulting partnerships, enhancing overall cloud data management efforts.
Compliance and Regulatory Standards
Organizations must ensure they follow rules based on the data they manage. Sensitive data often comes with strict guidelines. With different storage methods:
- Data Lakes: They may have flexible compliance needs but should closely monitor access to sensitive data.
- Data Warehouses: They must strictly comply with standards and are often subject to audits.
Insights into Governance Practices
As organizations grow, effective governance becomes very important. In data lakes, flexible governance is important for different data types. In contrast, data warehouses should have a structured governance plan focusing on data quality and compliance with regulations. Engaging in dwh consulting can enhance these governance strategies.
Integrating Data Lakes and Data Warehouses
Advantages of a Hybrid Data Strategy
Using both a data lake and a data warehouse can help organizations enjoy the benefits of both systems. This hybrid strategy allows businesses to explore raw data while also getting structured insights from reliable sources. Partnering through dwh consulting can facilitate this integration process effectively, improving overall data storage solutions.
Best Integration Practices
To effectively combine both systems, consider these practices:
- Build a Unified System: Create a management system that shows data between both environments, facilitating quick access.
- Institutionalize Data Integrity Checks: Regular checks ensure reliable and accurate data for analysis.
Identifying Challenges and Solutions
Maintaining synchronization between data lakes and warehouses can be challenging, leading to issues with data silos or inconsistencies. Solutions include having clear protocols for data classification and encouraging teamwork to align goals and improve integration. Input from dwh consulting can streamline overcoming these challenges.
Checklist for Choosing Between Data Lake and Data Warehouse
Criteria Evaluation
Before deciding, think about these criteria:
- Data Types: What kind of data does your organization need? Will it involve structured formats, semi-structured data, or unstructured content?
- Use Cases: How will you analyze and report this data? Is it mainly for real-time insights or structured reporting?
- Growth Projections: Forecast how fast your data environment might grow. Predicting this is vital. Consulting with dwh experts can shed light on projections and expectations.
Key Decision Factors
When picking between a data lake and a data warehouse, focus on these key aspects:
- Cost: Look at what your budget allows for data storage. What can you afford to spend upfront versus over time?
- Technology: Consider existing tools you need to keep. Compatibility can save hours of work.
- Speed Needs: Think about how quickly you need access to and analysis of your data. Knowing if you need immediate insights or structured reports can guide your choice.
Essential Questions for Stakeholders
Discuss these important questions with stakeholders before making a choice:
- What are our current and future data storage needs?
- How do we balance costs with performance needs?
- Which departments will benefit from each solution?
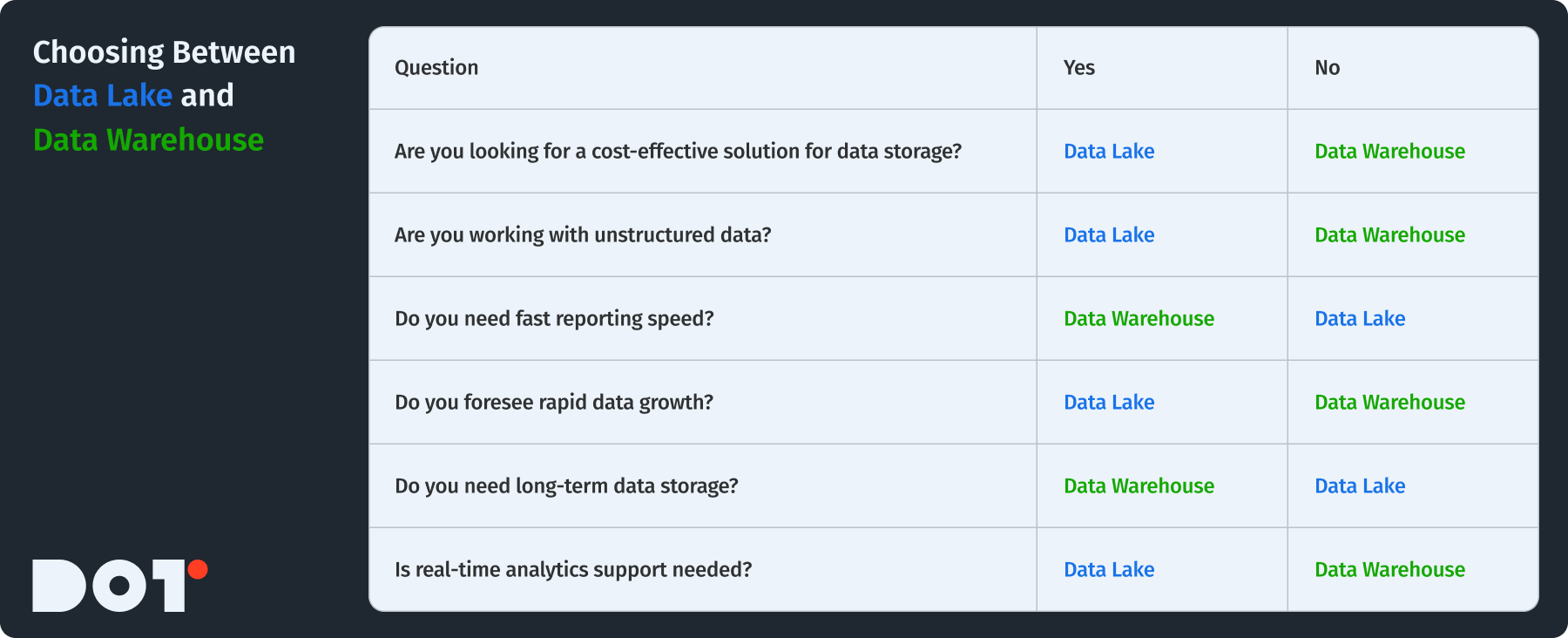
Summary
In summary, choosing between data lakes and data warehouses requires careful thought. Key differences include their definitions, structure, performance, security, and cost. Data lakes are quick and flexible, while data warehouses offer reliable structured data for better reporting and analysis. Each type serves unique purposes in different industries. Consulting services, particularly dwh consulting, can provide invaluable insights into these complexities. Additionally, leveraging data lake consulting can assist organizations in optimizing their data management processes even further.
If you still have questions and want specific advice, reach out to a Dot Analytics expert today for a free consultation. Having expert help can make your data management journey easier and more effective!